By borrowing techniques from astronomy, we can detect AI images and deep fakes.
According to a new study By the Royal Astronomical Society The AI-generated fakes could then be analyzed in the same way that astronomers study galaxies.
Adejumoke Owolabi, a master’s student at the University of Hull, concludes that it’s all down to reflections in people’s eyes: if the reflections match, the image is likely to be of a real person; if they don’t, it’s likely a deepfake.
“The eye reflections are consistent in the real person but are inaccurate (from a physics perspective) in the fake one,” explains Kevin Pimblett, professor of astrophysics at the University of Hull and director of the Centre of Excellence in Data Science, Artificial Intelligence and Modelling.
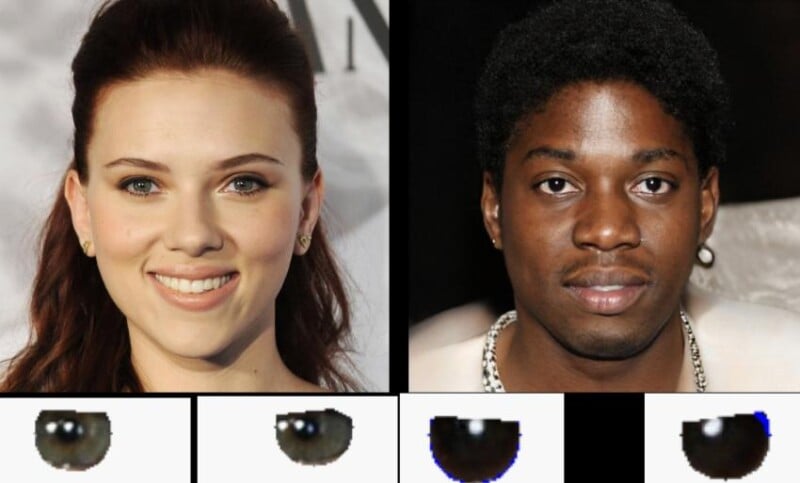
The researchers analyzed the light reflected by the human eye in real and AI-generated images, then borrowed a technique commonly used in astronomy to quantify the reflections and check for consistency between the left and right eyes.
“We measure the shape of a galaxy by analysing whether it has a compact centre, whether it has symmetry and how smooth it is – by analysing the distribution of light,” Prof Pimblett said.
“It automatically detects the reflections and passes their morphological characteristics through the CAS. [concentration, asymmetry, smoothness] We use the Gini coefficient to compare the similarity between the left and right eyeballs.
“Our findings suggest that there are some differences between the two types of deepfakes.”
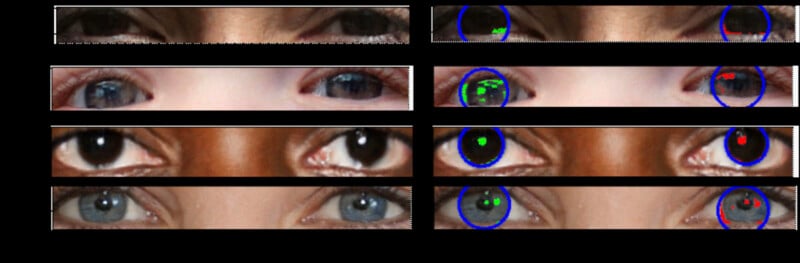
The Gini coefficient is typically used to measure how the light in an image of a galaxy is distributed across pixels, by ordering the pixels that make up the image of the galaxy in increasing order of flux, and comparing the result to what would be expected from a perfectly uniform flux distribution.
A Gini value of 0 is a galaxy whose light is evenly distributed across all pixels in the image, and a Gini value of 1 is a galaxy whose light is all concentrated in one pixel.

“It’s important to note that this is not a silver bullet for detecting fake images,” Professor Pimblett says.
“There will be false positives and false negatives, and it won’t catch everything, but this method gives us a basis, a plan of attack, in the arms race to detect deepfakes.”
Image credits: Royal Astronomical Society.