Getty Images
In ancient Chinese games goState-of-the-art artificial intelligence was generally able to beat the best human players At least since 2016But in recent years, researchers Flaws found in these top-level AIs go algorithm that Give humanity a fighting chanceBy using unorthodox “cyclical” strategies – strategies that even novice humans can detect and defeat – crafty humans can exploit gaps in the strategies of top-level AI, tricking the algorithm and causing it to lose.
With MIT researchers Fur AI They wanted to see whether they could improve on this “worst” performance in an otherwise “superhuman” AI Go algorithm, so they tested three ways of strengthening the top-level algorithm. KataGo algorithmDefending against adversarial attacks.,The results show that creating a truly robust and,unexploitable AI can be difficult, even in a,domain as tightly controlled as board games.
Three strategies that failed
In the preprint paper “can go Will AI become adversarially robust?The researchers go AI that is truly “robust” against all attacks – that is, not one that makes “game-losing blunders” that humans wouldn’t make, but one that requires competing AI algorithms to expend significant computing resources to beat it. Ideally, a robust algorithm should be able to use additional computing resources when faced with unknown situations to overcome potential attacks.
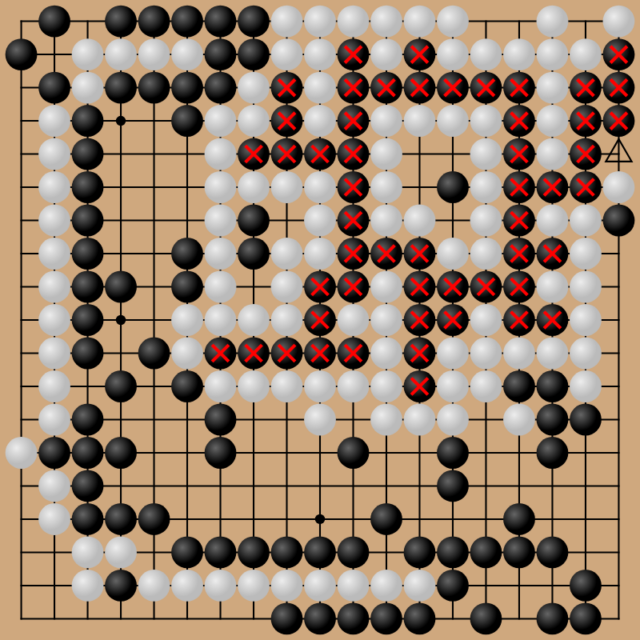
The researchers tried three methods to generate such robust data. go Algorithm. In the first stage, the researchers simply tweaked the KataGo model with more examples of unorthodox patrol strategies that had previously beaten the KataGo model, in the hope that by seeing more patterns, KataGo could learn to detect and beat these patterns.
This strategy initially looked promising, enabling KataGo to win 100 percent of its games against a periodic “attacker.” But after the attacker itself was tweaked (a process that used much less computing power than KataGo’s tweaks), its win rate dropped to 9 percent against small variations on the original attack.
In the second defense attempt, the researchers repeated multiple rounds of an “arms race,” in which new adversarial models discovered new exploits and new defense models attempted to plug those newly discovered holes. Even after 10 rounds of such iterative training, the final defense algorithm only managed to win 19 percent of games against the final attack algorithm, which had discovered never-before-seen variations of the exploit. This was true even when the updated algorithm maintained an advantage over the previous attacker that had been trained in the past.
Getty Images
As a final attempt, the researchers Vision TransformerThis is an attempt to avoid the “bad inductive biases” present in the convolutional neural network that originally trained KataGo. This method also failed, winning only 22 percent of the time against variations of a patrol attack that were “reproducible by human experts,” the researchers wrote.
Will it have any effect?
In all three defense attempts, the opponents who defeated KataGo generally did not represent new heights previously unseen. goInstead, these attack algorithms focused on finding exploitable weaknesses in high-performing AI algorithms, even though they would lose to most human players using simple attack strategies.
These exploitable holes highlight the importance of evaluating the “worst-case” performance of an AI system, even if its “average” performance seems superhuman. On average, KataGo can beat even high-level human players using traditional strategies. But in the worst case, an otherwise “weak” opponent can find a hole in the system and cause it to collapse.
It’s easy to extend this thinking to other types of generative AI systems. Successfully complete several complex creative and referential tasks Maybe not yet When faced with a trivial math problem, they fail completely (or Being “poisoned” by malicious prompts). Visual AI models Explain and analyze complex photographs nevertheless Fail miserably when presented with basic geometric shapes.
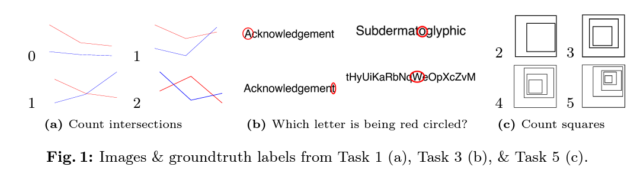
Improving these “worst case” scenarios is Avoid embarrassing mistakes When releasing AI systems to the public, it is often much quicker and easier for a determined “adversary” to find new holes in an AI algorithm’s performance than it is to improve the algorithm and fix the issues, the new research finds.
And if that’s true goThat may be even more true in uncontrolled environments, in games that are highly complex but have tightly defined rules. “The thing about AI is that these vulnerabilities are hard to eliminate,” said Adam Grieve, CEO of FAR. He told Nature“If you can’t solve the problem in a simple domain, go,If this is the case, there seems to be little prospect of a short-term fix for similar issues like the ChatGPT jailbreak.”
Still, the researchers are not despairing. None of their methods ” [new] Attack is impossible.” goTheir strategy was able to plug previously identified, immutable, “fixed” exploits. This is because go “The AI can be hardened by training it against a large enough set of attacks,” the researchers write, proposing future work that could achieve this.
Either way, this new research shows that making AI systems more robust against worst-case scenarios may be just as valuable as pursuing new, more human/superhuman capabilities.